Spaces:
Runtime error
Runtime error
File size: 18,374 Bytes
eadd7b4 |
1 2 3 4 5 6 7 8 9 10 11 12 13 14 15 16 17 18 19 20 21 22 23 24 25 26 27 28 29 30 31 32 33 34 35 36 37 38 39 40 41 42 43 44 45 46 47 48 49 50 51 52 53 54 55 56 57 58 59 60 61 62 63 64 65 66 67 68 69 70 71 72 73 74 75 76 77 78 79 80 81 82 83 84 85 86 87 88 89 90 91 92 93 94 95 96 97 98 99 100 101 102 103 104 105 106 107 108 109 110 111 112 113 114 115 116 117 118 119 120 121 122 123 124 125 126 127 128 129 130 131 132 133 134 135 136 137 138 139 140 141 142 143 144 145 146 147 148 149 150 151 152 153 154 155 156 157 158 159 160 161 162 163 164 165 166 167 168 169 170 171 172 173 174 175 176 177 178 179 180 181 182 183 184 185 186 187 188 189 190 191 192 193 194 195 196 197 198 199 200 201 202 203 204 205 206 207 208 209 210 211 212 213 214 215 216 217 218 219 220 221 222 223 224 225 226 227 228 229 230 231 232 233 234 235 236 237 238 239 240 241 242 243 244 245 246 247 248 249 250 251 252 253 254 255 256 257 258 259 260 261 262 263 264 265 266 267 268 269 270 271 272 273 274 275 276 277 278 279 280 281 282 283 284 285 286 287 288 289 290 291 292 293 294 295 296 297 298 299 300 301 302 303 304 305 306 307 308 309 310 311 312 313 314 315 316 317 318 319 320 321 322 323 324 325 326 327 328 329 330 331 332 333 334 335 336 337 338 339 340 341 342 343 344 345 346 347 348 349 350 351 352 353 354 355 356 357 358 359 360 361 362 363 364 365 366 367 368 369 370 371 372 373 374 375 376 377 378 379 380 381 382 383 384 385 386 387 388 389 390 391 392 393 394 395 396 397 398 399 400 401 402 403 404 405 406 407 408 409 410 411 412 413 414 415 416 417 418 419 420 421 |
#!/usr/bin/env python
from __future__ import annotations
import argparse
import os
import sys
from pathlib import Path
current_file_path = Path(__file__).resolve()
sys.path.insert(0, str(current_file_path.parent.parent))
import random
import gradio as gr
import numpy as np
import uuid
from diffusers import ConsistencyDecoderVAE, DPMSolverMultistepScheduler, Transformer2DModel, AutoencoderKL
import torch
from typing import Tuple
from datetime import datetime
from diffusion.sa_solver_diffusers import SASolverScheduler
from peft import PeftModel
from scripts.diffusers_patches import pixart_sigma_init_patched_inputs, PixArtSigmaPipeline
DESCRIPTION = """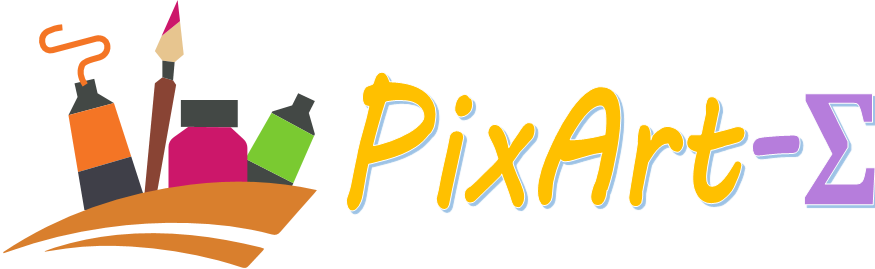
# PixArt-Sigma 1024px
#### [PixArt-Sigma 1024px](https://github.com/PixArt-alpha/PixArt-sigma) is a transformer-based text-to-image diffusion system trained on text embeddings from T5. This demo uses the [PixArt-alpha/PixArt-XL-2-1024-MS](https://huggingface.co/PixArt-alpha/PixArt-XL-2-1024-MS) checkpoint.
#### English prompts ONLY; 提示词仅限英文
### <span style='color: red;'>You may change the DPM-Solver inference steps from 14 to 20, or DPM-Solver Guidance scale from 4.5 to 3.5 if you didn't get satisfied results.
"""
if not torch.cuda.is_available():
DESCRIPTION += "\n<p>Running on CPU 🥶 This demo does not work on CPU.</p>"
MAX_SEED = np.iinfo(np.int32).max
CACHE_EXAMPLES = torch.cuda.is_available() and os.getenv("CACHE_EXAMPLES", "1") == "1"
MAX_IMAGE_SIZE = int(os.getenv("MAX_IMAGE_SIZE", "6000"))
USE_TORCH_COMPILE = os.getenv("USE_TORCH_COMPILE", "0") == "1"
ENABLE_CPU_OFFLOAD = os.getenv("ENABLE_CPU_OFFLOAD", "0") == "1"
PORT = int(os.getenv("DEMO_PORT", "15432"))
device = torch.device("cuda:0" if torch.cuda.is_available() else "cpu")
style_list = [
{
"name": "(No style)",
"prompt": "{prompt}",
"negative_prompt": "",
},
{
"name": "Cinematic",
"prompt": "cinematic still {prompt} . emotional, harmonious, vignette, highly detailed, high budget, bokeh, cinemascope, moody, epic, gorgeous, film grain, grainy",
"negative_prompt": "anime, cartoon, graphic, text, painting, crayon, graphite, abstract, glitch, deformed, mutated, ugly, disfigured",
},
{
"name": "Photographic",
"prompt": "cinematic photo {prompt} . 35mm photograph, film, bokeh, professional, 4k, highly detailed",
"negative_prompt": "drawing, painting, crayon, sketch, graphite, impressionist, noisy, blurry, soft, deformed, ugly",
},
{
"name": "Anime",
"prompt": "anime artwork {prompt} . anime style, key visual, vibrant, studio anime, highly detailed",
"negative_prompt": "photo, deformed, black and white, realism, disfigured, low contrast",
},
{
"name": "Manga",
"prompt": "manga style {prompt} . vibrant, high-energy, detailed, iconic, Japanese comic style",
"negative_prompt": "ugly, deformed, noisy, blurry, low contrast, realism, photorealistic, Western comic style",
},
{
"name": "Digital Art",
"prompt": "concept art {prompt} . digital artwork, illustrative, painterly, matte painting, highly detailed",
"negative_prompt": "photo, photorealistic, realism, ugly",
},
{
"name": "Pixel art",
"prompt": "pixel-art {prompt} . low-res, blocky, pixel art style, 8-bit graphics",
"negative_prompt": "sloppy, messy, blurry, noisy, highly detailed, ultra textured, photo, realistic",
},
{
"name": "Fantasy art",
"prompt": "ethereal fantasy concept art of {prompt} . magnificent, celestial, ethereal, painterly, epic, majestic, magical, fantasy art, cover art, dreamy",
"negative_prompt": "photographic, realistic, realism, 35mm film, dslr, cropped, frame, text, deformed, glitch, noise, noisy, off-center, deformed, cross-eyed, closed eyes, bad anatomy, ugly, disfigured, sloppy, duplicate, mutated, black and white",
},
{
"name": "Neonpunk",
"prompt": "neonpunk style {prompt} . cyberpunk, vaporwave, neon, vibes, vibrant, stunningly beautiful, crisp, detailed, sleek, ultramodern, magenta highlights, dark purple shadows, high contrast, cinematic, ultra detailed, intricate, professional",
"negative_prompt": "painting, drawing, illustration, glitch, deformed, mutated, cross-eyed, ugly, disfigured",
},
{
"name": "3D Model",
"prompt": "professional 3d model {prompt} . octane render, highly detailed, volumetric, dramatic lighting",
"negative_prompt": "ugly, deformed, noisy, low poly, blurry, painting",
},
]
styles = {k["name"]: (k["prompt"], k["negative_prompt"]) for k in style_list}
STYLE_NAMES = list(styles.keys())
DEFAULT_STYLE_NAME = "(No style)"
SCHEDULE_NAME = ["DPM-Solver", "SA-Solver"]
DEFAULT_SCHEDULE_NAME = "DPM-Solver"
NUM_IMAGES_PER_PROMPT = 1
def apply_style(style_name: str, positive: str, negative: str = "") -> Tuple[str, str]:
p, n = styles.get(style_name, styles[DEFAULT_STYLE_NAME])
if not negative:
negative = ""
return p.replace("{prompt}", positive), n + negative
def get_args():
parser = argparse.ArgumentParser()
parser.add_argument('--is_lora', action='store_true', help='enable lora ckpt loading')
parser.add_argument('--repo_id', default="PixArt-alpha/PixArt-Sigma-XL-2-1024-MS", type=str)
parser.add_argument('--lora_repo_id', default=None, type=str)
parser.add_argument('--model_path', default=None, type=str)
parser.add_argument(
'--pipeline_load_from', default="PixArt-alpha/pixart_sigma_sdxlvae_T5_diffusers", type=str,
help="Download for loading text_encoder, tokenizer and vae "
"from https://huggingface.co/PixArt-alpha/PixArt-XL-2-1024-MS")
parser.add_argument('--T5_token_max_length', default=120, type=int, help='max length of tokens for T5')
return parser.parse_args()
args = get_args()
if torch.cuda.is_available():
weight_dtype = torch.float16
T5_token_max_length = args.T5_token_max_length
model_path = args.model_path
if 'Sigma' in args.model_path:
T5_token_max_length = 300
# tmp patches for diffusers PixArtSigmaPipeline Implementation
print(
"Changing _init_patched_inputs method of diffusers.models.Transformer2DModel "
"using scripts.diffusers_patches.pixart_sigma_init_patched_inputs")
setattr(Transformer2DModel, '_init_patched_inputs', pixart_sigma_init_patched_inputs)
if not args.is_lora:
transformer = Transformer2DModel.from_pretrained(
model_path,
subfolder='transformer',
torch_dtype=weight_dtype,
)
pipe = PixArtSigmaPipeline.from_pretrained(
args.pipeline_load_from,
transformer=transformer,
torch_dtype=weight_dtype,
use_safetensors=True,
)
else:
assert args.lora_repo_id is not None
transformer = Transformer2DModel.from_pretrained(args.repo_id, subfolder="transformer", torch_dtype=torch.float16)
transformer = PeftModel.from_pretrained(transformer, args.lora_repo_id)
pipe = PixArtSigmaPipeline.from_pretrained(
args.repo_id,
transformer=transformer,
torch_dtype=torch.float16,
use_safetensors=True,
)
del transformer
if os.getenv('CONSISTENCY_DECODER', False):
print("Using DALL-E 3 Consistency Decoder")
pipe.vae = ConsistencyDecoderVAE.from_pretrained("openai/consistency-decoder", torch_dtype=torch.float16)
if ENABLE_CPU_OFFLOAD:
pipe.enable_model_cpu_offload()
else:
pipe.to(device)
print("Loaded on Device!")
# speed-up T5
pipe.text_encoder.to_bettertransformer()
if USE_TORCH_COMPILE:
pipe.transformer = torch.compile(pipe.transformer, mode="reduce-overhead", fullgraph=True)
print("Model Compiled!")
def save_image(img, seed=''):
unique_name = f"{str(uuid.uuid4())}_{seed}.png"
save_path = os.path.join(f'output/online_demo_img/{datetime.now().date()}')
os.umask(0o000) # file permission: 666; dir permission: 777
os.makedirs(save_path, exist_ok=True)
unique_name = os.path.join(save_path, unique_name)
img.save(unique_name)
return unique_name
def randomize_seed_fn(seed: int, randomize_seed: bool) -> int:
if randomize_seed:
seed = random.randint(0, MAX_SEED)
return seed
@torch.no_grad()
@torch.inference_mode()
def generate(
prompt: str,
negative_prompt: str = "",
style: str = DEFAULT_STYLE_NAME,
use_negative_prompt: bool = False,
num_imgs: int = 1,
seed: int = 0,
width: int = 1024,
height: int = 1024,
schedule: str = 'DPM-Solver',
dpms_guidance_scale: float = 4.5,
sas_guidance_scale: float = 3,
dpms_inference_steps: int = 20,
sas_inference_steps: int = 25,
randomize_seed: bool = False,
use_resolution_binning: bool = True,
progress=gr.Progress(track_tqdm=True),
):
seed = int(randomize_seed_fn(seed, randomize_seed))
generator = torch.Generator().manual_seed(seed)
print(f"{PORT}: {model_path}")
print(prompt)
if schedule == 'DPM-Solver':
if not isinstance(pipe.scheduler, DPMSolverMultistepScheduler):
pipe.scheduler = DPMSolverMultistepScheduler()
num_inference_steps = dpms_inference_steps
guidance_scale = dpms_guidance_scale
elif schedule == "SA-Solver":
if not isinstance(pipe.scheduler, SASolverScheduler):
pipe.scheduler = SASolverScheduler.from_config(pipe.scheduler.config, algorithm_type='data_prediction', tau_func=lambda t: 1 if 200 <= t <= 800 else 0, predictor_order=2, corrector_order=2)
num_inference_steps = sas_inference_steps
guidance_scale = sas_guidance_scale
else:
raise ValueError(f"Unknown schedule: {schedule}")
if not use_negative_prompt:
negative_prompt = None # type: ignore
prompt, negative_prompt = apply_style(style, prompt, negative_prompt)
images = pipe(
prompt=prompt,
width=width,
height=height,
guidance_scale=guidance_scale,
num_inference_steps=num_inference_steps,
generator=generator,
num_images_per_prompt=num_imgs,
use_resolution_binning=use_resolution_binning,
output_type="pil",
max_sequence_length=args.T5_token_max_length,
).images
image_paths = [save_image(img, seed) for img in images]
print(image_paths)
return image_paths, seed
examples = [
"A small cactus with a happy face in the Sahara desert.",
"an astronaut sitting in a diner, eating fries, cinematic, analog film",
"Pirate ship trapped in a cosmic maelstrom nebula, rendered in cosmic beach whirlpool engine, volumetric lighting, spectacular, ambient lights, light pollution, cinematic atmosphere, art nouveau style, illustration art artwork by SenseiJaye, intricate detail.",
"stars, water, brilliantly, gorgeous large scale scene, a little girl, in the style of dreamy realism, light gold and amber, blue and pink, brilliantly illuminated in the background.",
"professional portrait photo of an anthropomorphic cat wearing fancy gentleman hat and jacket walking in autumn forest.",
"beautiful lady, freckles, big smile, blue eyes, short ginger hair, dark makeup, wearing a floral blue vest top, soft light, dark grey background",
"Spectacular Tiny World in the Transparent Jar On the Table, interior of the Great Hall, Elaborate, Carved Architecture, Anatomy, Symetrical, Geometric and Parameteric Details, Precision Flat line Details, Pattern, Dark fantasy, Dark errie mood and ineffably mysterious mood, Technical design, Intricate Ultra Detail, Ornate Detail, Stylized and Futuristic and Biomorphic Details, Architectural Concept, Low contrast Details, Cinematic Lighting, 8k, by moebius, Fullshot, Epic, Fullshot, Octane render, Unreal ,Photorealistic, Hyperrealism",
"anthropomorphic profile of the white snow owl Crystal priestess , art deco painting, pretty and expressive eyes, ornate costume, mythical, ethereal, intricate, elaborate, hyperrealism, hyper detailed, 3D, 8K, Ultra Realistic, high octane, ultra resolution, amazing detail, perfection, In frame, photorealistic, cinematic lighting, visual clarity, shading , Lumen Reflections, Super-Resolution, gigapixel, color grading, retouch, enhanced, PBR, Blender, V-ray, Procreate, zBrush, Unreal Engine 5, cinematic, volumetric, dramatic, neon lighting, wide angle lens ,no digital painting blur",
"The parametric hotel lobby is a sleek and modern space with plenty of natural light. The lobby is spacious and open with a variety of seating options. The front desk is a sleek white counter with a parametric design. The walls are a light blue color with parametric patterns. The floor is a light wood color with a parametric design. There are plenty of plants and flowers throughout the space. The overall effect is a calm and relaxing space. occlusion, moody, sunset, concept art, octane rendering, 8k, highly detailed, concept art, highly detailed, beautiful scenery, cinematic, beautiful light, hyperreal, octane render, hdr, long exposure, 8K, realistic, fog, moody, fire and explosions, smoke, 50mm f2.8",
]
with gr.Blocks(css="scripts/style.css") as demo:
gr.Markdown(DESCRIPTION)
gr.DuplicateButton(
value="Duplicate Space for private use",
elem_id="duplicate-button",
visible=os.getenv("SHOW_DUPLICATE_BUTTON") == "1",
)
with gr.Row(equal_height=False):
with gr.Group():
with gr.Row():
prompt = gr.Text(
label="Prompt",
show_label=False,
max_lines=1,
placeholder="Enter your prompt",
container=False,
)
run_button = gr.Button("Run", scale=0)
result = gr.Gallery(label="Result", show_label=False)
# with gr.Accordion("Advanced options", open=False):
with gr.Group():
with gr.Row():
use_negative_prompt = gr.Checkbox(label="Use negative prompt", value=False, visible=True)
with gr.Row(visible=True):
schedule = gr.Radio(
show_label=True,
container=True,
interactive=True,
choices=SCHEDULE_NAME,
value=DEFAULT_SCHEDULE_NAME,
label="Sampler Schedule",
visible=True,
)
num_imgs = gr.Slider(
label="Num Images",
minimum=1,
maximum=8,
step=1,
value=1,
)
style_selection = gr.Radio(
show_label=True,
container=True,
interactive=True,
choices=STYLE_NAMES,
value=DEFAULT_STYLE_NAME,
label="Image Style",
)
negative_prompt = gr.Text(
label="Negative prompt",
max_lines=1,
placeholder="Enter a negative prompt",
visible=True,
)
seed = gr.Slider(
label="Seed",
minimum=0,
maximum=MAX_SEED,
step=1,
value=0,
)
randomize_seed = gr.Checkbox(label="Randomize seed", value=True)
with gr.Row(visible=True):
width = gr.Slider(
label="Width",
minimum=256,
maximum=MAX_IMAGE_SIZE,
step=32,
value=1024,
)
height = gr.Slider(
label="Height",
minimum=256,
maximum=MAX_IMAGE_SIZE,
step=32,
value=1024,
)
with gr.Row():
dpms_guidance_scale = gr.Slider(
label="DPM-Solver Guidance scale",
minimum=1,
maximum=10,
step=0.1,
value=4.5,
)
dpms_inference_steps = gr.Slider(
label="DPM-Solver inference steps",
minimum=5,
maximum=40,
step=1,
value=14,
)
with gr.Row():
sas_guidance_scale = gr.Slider(
label="SA-Solver Guidance scale",
minimum=1,
maximum=10,
step=0.1,
value=3,
)
sas_inference_steps = gr.Slider(
label="SA-Solver inference steps",
minimum=10,
maximum=40,
step=1,
value=25,
)
gr.Examples(
examples=examples,
inputs=prompt,
outputs=[result, seed],
fn=generate,
cache_examples=CACHE_EXAMPLES,
)
use_negative_prompt.change(
fn=lambda x: gr.update(visible=x),
inputs=use_negative_prompt,
outputs=negative_prompt,
api_name=False,
)
gr.on(
triggers=[
prompt.submit,
negative_prompt.submit,
run_button.click,
],
fn=generate,
inputs=[
prompt,
negative_prompt,
style_selection,
use_negative_prompt,
num_imgs,
seed,
width,
height,
schedule,
dpms_guidance_scale,
sas_guidance_scale,
dpms_inference_steps,
sas_inference_steps,
randomize_seed,
],
outputs=[result, seed],
api_name="run",
)
if __name__ == "__main__":
demo.queue(max_size=20).launch(server_name="0.0.0.0", server_port=PORT, debug=True)
|